Back when I worked for Capital One, I was given a short term assignment to join a team of fellow business analysts and statisticians to rebuild one of our Subprime credit models. We used tools like SQL, SAS, and CART to identify the variables that were most highly correlated with payments, profit, and losses. While the math work was pretty individualistic, we often consulted with each other to check our intuition and ensure we were building a robust model.
We kicked off the project with a team meeting, and we had a very strong team. Gary lead the team – he was eagerly anticipating a promotion, and was hoping that leading this project would solidify his chances at the next review period. HJ was the lead statistician. I had worked with her before on a collections and recoveries model and she was fantastic to work with – she was one of the few statisticians who could take her head out of the numbers and evaluate the bigger picture of the work. And rounding out the team was Mike, who was a tremendously hard worker and had just got done building another credit model, so he was in the right frame of mind.
My task was to re-build the late payment fee portion of the model. I didn’t want to cloud my judgement, so I didn’t even look at what variables were used previously so I could attack the problem objectively. I ran the data through CART, picked the best variables, and ran them through SAS to get an initial working model. Being the mid-2000s, mortgage data was very highly correlated to good credit risk customers. This was not surprising for the time – house prices were booming, and Subprime customers were paying back credit cards with cash-out refinancings – a cash source that was soon to dry up.
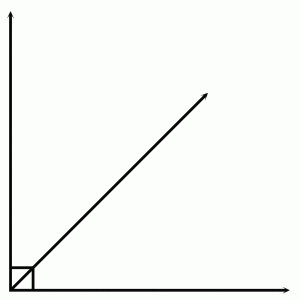
hypothesis – impact of late fee amount
I consulted with HJ on my findings, and we decided to remove many of the mortgage variables to remove bias from the model (a decision that would prove wise and beneficial for Capital One as the mortgage market melted down and Capital One emerged as perhaps the most resilient consumer credit company in the nation). When we felt confident about the revised model, we used backtesting to ensure the model performed well – i.e. used sample data to see if the model actually worked.
I was quite surprised with the results from the model and backtesting: a higher late payment fee didn’t necessarily equal greater profits (or even greater revenue).
I had anticipated the late payment fee impact would be close to linear – the higher the fee, the more revenue and profit. I figured the more we could charge, the better, and the main constraint would be that customers wouldn’t sign up for a card with a high late payment fee (these were the days before the CARD Act that capped the late fee). My hypothesis was that raising the fee would increase the profits from any particular customer, but may drive a decrease in the number of total customers.
HJ and I found out, though that the amount of the late fee has a parabolic impact on profits at a customer level. There’s a tipping point, and once the fee exceeds that amount, profit actually declines. The fee becomes so onerous that customers stop paying their bills, resulting in losses for Capital One. Again, these were Subprime borrowers, many teetering on the brink of default anyway, and a $39 late fee vs. $29 could be the difference between solvency and default. But it’s a stark difference to my hypothesis – that raising the late fee can create capital losses.
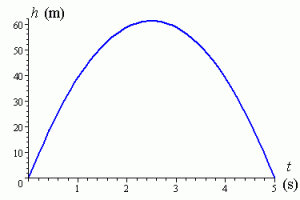
Actual – impact of late fee amount
The end result was that we didn’t use the highest late fee in all circumstances. For some borrowers, it was better to actually have a lower late fee. And, it brought about a new credit policy on late fee waivers – Capital One led the charge in proactively and re-actively waiving late fees, both to drive loyalty as well as reduce losses.
Gary was very happy with the result of the model and the impact on the business. He got his promotion, and ended up leaving Capital One a short time later for another step-up. Mike had the unenviable task of splicing the various pieces of the model together, and worked hard and kept his smile on his face. HJ moved on to the next big model needing a rebuild. An I went back to customer acquisitions and portfolio management.
But there was another, meta-takeaway I took with me from the experience – the law of unintended consequences, particularly around credit risk. Higher fees and interest don’t always yield higher profits.
It’s one of the reason at Peer Lending Advisors we look at both the interest rate (expected revenue) and risk of loss (expected capital loss) to create our model for expected loan profitability. Chasing returns is for fools – if we don’t think we can make a fair return on our capital, we sit on the sidelines. And we tread carefully where interest rates are above “D” grade, knowing the risks of customers in these classes.
Interested in leveraging the experience of Peer Lending Advisors to help guide you through Peer-to-Peer Lending? Call (425-246-5436) or drop a line – Peer Lending Advisors would love to work with you.
[contact-form][contact-field label=’Name’ type=’name’ required=’1’/][contact-field label=’Email’ type=’email’ required=’1’/][contact-field label=’Phone’ type=’text’/][/contact-form]